-
Notifications
You must be signed in to change notification settings - Fork 66
Commit
This commit does not belong to any branch on this repository, and may belong to a fork outside of the repository.
* Add summary * Added the conference name and the year of publication
- Loading branch information
1 parent
25837cd
commit 9ab62eb
Showing
2 changed files
with
94 additions
and
0 deletions.
There are no files selected for viewing
This file contains bidirectional Unicode text that may be interpreted or compiled differently than what appears below. To review, open the file in an editor that reveals hidden Unicode characters.
Learn more about bidirectional Unicode characters
Original file line number | Diff line number | Diff line change |
---|---|---|
@@ -0,0 +1,39 @@ | ||
# Cycle-Dehaze: Enhanced CycleGAN for Single Image Dehazing | ||
|
||
Deniz Engin, Anıl Genc, Hazım Kemal Ekenel | ||
|
||
## Summary | ||
|
||
This paper proposes an end-to-end network for single-image dehazing problems, which is an enhanced version of the cycleGAN method, i.e, this also works on unpaired images in the datasets. The provided method is better than the state of the art methods for single-image dehazing which use the atmospheric scattering model. | ||
|
||
## Main contributions | ||
|
||
- The addition of perceptual cycle loss in the original cycleGAN architecture, which compares images in a feature space rather than the pixel space. | ||
|
||
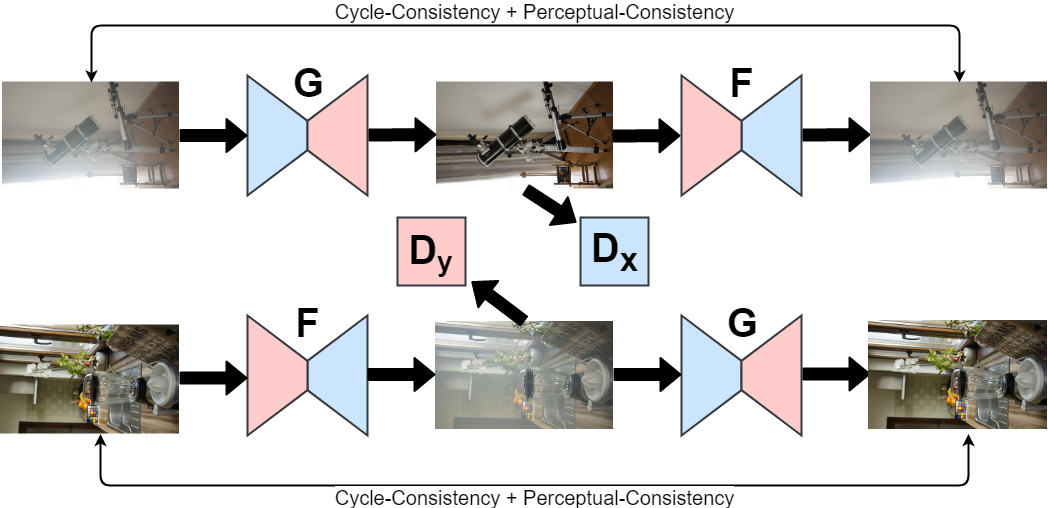 | ||
|
||
- Since the paper is adopting the original cycleGAN architecture, hence dataset of unpaired images is used. | ||
|
||
- This method does not rely on calculating the parameters of the atmospheric scattering model (which is used in the earlier methods). | ||
|
||
- To upscale the output images, Laplacian pyramid is used instead of the bicubic-upscaling method to get a higher resolution and sharp image for better haze free images. | ||
|
||
- Due to its cyclic structure, the model turns out to be generalized and can be used for other datasets. | ||
|
||
## Implementation details | ||
|
||
- The CycleGAN model architecture is adapted from the original [CycleGAN](https://arxiv.org/pdf/1703.10593.pdf) paper, with the addition of a cycle perceptual loss inspired by the [EnhanceNet paper](https://arxiv.org/pdf/1612.07919.pdf). To achieve high-resolution output images, the model utilizes the [Laplacian pyramid](https://arxiv.org/pdf/1506.05751.pdf) method. | ||
|
||
## Two-cents | ||
|
||
- The model achieves very significant results even in the absence of the ground-truth images, unlike the state of the art methods. | ||
|
||
- The is a generalizable model as it learns the dehazing task rather than overfitting on the data. | ||
|
||
- Higher weightage is given to cycle consistency loss as compared to perceptual cycle loss, as higher weightage to the perceptual cycle loss causes loss of color information after dehazing. | ||
|
||
## Resources | ||
|
||
- Paper:- https://arxiv.org/pdf/1805.05308.pdf | ||
|
||
- Implementation:- https://github.com/engindeniz/Cycle-Dehaze/ |
This file contains bidirectional Unicode text that may be interpreted or compiled differently than what appears below. To review, open the file in an editor that reveals hidden Unicode characters.
Learn more about bidirectional Unicode characters
Original file line number | Diff line number | Diff line change |
---|---|---|
@@ -0,0 +1,55 @@ | ||
# A Style-Based Generator Architecture for Generative Adversarial Networks | ||
|
||
- Authors:- Tero Karras, Samuli Laine, Timo Aila | ||
- Conference name:- The IEEE Conference on Computer Vision and Pattern Recognition (CVPR) | ||
- Year of publication:- 2018 | ||
|
||
## Summary | ||
|
||
This paper proposes a generative architecture, which is an enhanced version of proGAN, which helps in unsupervised separation of the high-level attributes(like pose and identity in humans) and of stochastic variation in the generated images(like hairs, etc.). The proposed method generates images of very high quality(like 1024x1024) and also improves the disentanglement of the latent vectors of variation. | ||
|
||
## Main contributions | ||
|
||
- Replace the nearest-neighbour up/downsampling in both networks with bilinear sampling. | ||
|
||
- Instead of giving input as a latent vector, a learned constant input is given and at each convolution layer, a latent vector(w) is given to adjust the styles of the image. | ||
|
||
- The latent vector(w) is obtained by a non-linear mapping from a random generated latent vector(z) using 8 layer MLP. | ||
w is then passed to affine transformation which helps to control adaptive instance normalization (AdaIN) operations after each convolution layer. The AdaIn replaces the pixel norm that was used in the original proGAN architecture. | ||
|
||
- Gaussian noise is injected after every convolution layer, which helps in the unsupervised separation of the high-level attributes and the stochastic variation. | ||
|
||
- Use of mixing regularization(using two latent vectoers instead of one) for increasing the localisation of the styles , which increases the FID score. | ||
|
||
- Proposal of two new metrics for the evaluating disentanglement, which do not require an encoder network :-> perceptual path-length and linear separability | ||
|
||
- A new dataset of human faces(Flickr-Faces-HQ, FFHQ) with much higher quality and diversity is presented. | ||
|
||
## Generator network architecture | ||
|
||
 | ||
|
||
## Implementation details | ||
|
||
- The generator architecture is modified from the generator architecture of the original [proGAN](https://arxiv.org/pdf/1710.10196v3.pdf) paper. | ||
|
||
## Two-cent | ||
|
||
- The main idea of the proposed paper is to get a better control over the image synthesis process. | ||
|
||
- The mapping network and the affine tramsformations draw samples for each style from a learned distribution, while the synthesis network generates images based on collection of those styles. | ||
|
||
- There is no modification in the discriminator architecture and the loss functions of proGAN, the major change is in the generator architecture. | ||
|
||
- The collection of styles(in human faces) is like | ||
- Coarse styles(4x4 - 8x8) :-> pose, hair, face shape | ||
- Middle styles(16x16 - 32x32) :-> facial features, eyes | ||
- Fine styles(64x64 - 1024x1024) :-> color scheme | ||
|
||
## Resources | ||
|
||
- Paper :- https://arxiv.org/pdf/1812.04948.pdf | ||
|
||
- Demonstrative Video :- https://youtu.be/kSLJriaOumA | ||
|
||
- Implementation :- https://github.com/NVlabs/stylegan |