-
Notifications
You must be signed in to change notification settings - Fork 0
/
Copy pathapp.py
504 lines (374 loc) · 22.8 KB
/
app.py
1
2
3
4
5
6
7
8
9
10
11
12
13
14
15
16
17
18
19
20
21
22
23
24
25
26
27
28
29
30
31
32
33
34
35
36
37
38
39
40
41
42
43
44
45
46
47
48
49
50
51
52
53
54
55
56
57
58
59
60
61
62
63
64
65
66
67
68
69
70
71
72
73
74
75
76
77
78
79
80
81
82
83
84
85
86
87
88
89
90
91
92
93
94
95
96
97
98
99
100
101
102
103
104
105
106
107
108
109
110
111
112
113
114
115
116
117
118
119
120
121
122
123
124
125
126
127
128
129
130
131
132
133
134
135
136
137
138
139
140
141
142
143
144
145
146
147
148
149
150
151
152
153
154
155
156
157
158
159
160
161
162
163
164
165
166
167
168
169
170
171
172
173
174
175
176
177
178
179
180
181
182
183
184
185
186
187
188
189
190
191
192
193
194
195
196
197
198
199
200
201
202
203
204
205
206
207
208
209
210
211
212
213
214
215
216
217
218
219
220
221
222
223
224
225
226
227
228
229
230
231
232
233
234
235
236
237
238
239
240
241
242
243
244
245
246
247
248
249
250
251
252
253
254
255
256
257
258
259
260
261
262
263
264
265
266
267
268
269
270
271
272
273
274
275
276
277
278
279
280
281
282
283
284
285
286
287
288
289
290
291
292
293
294
295
296
297
298
299
300
301
302
303
304
305
306
307
308
309
310
311
312
313
314
315
316
317
318
319
320
321
322
323
324
325
326
327
328
329
330
331
332
333
334
335
336
337
338
339
340
341
342
343
344
345
346
347
348
349
350
351
352
353
354
355
356
357
358
359
360
361
362
363
364
365
366
367
368
369
370
371
372
373
374
375
376
377
378
379
380
381
382
383
384
385
386
387
388
389
390
391
392
393
394
395
396
397
398
399
400
401
402
403
404
405
406
407
408
409
410
411
412
413
414
415
416
417
418
419
420
421
422
423
424
425
426
427
428
429
430
431
432
433
434
435
436
437
438
439
440
441
442
443
444
445
446
447
448
449
450
451
452
453
454
455
456
457
458
459
460
461
462
463
464
465
466
467
468
469
470
471
472
473
474
475
476
477
478
479
480
481
482
483
484
485
486
487
488
489
490
491
492
493
494
495
496
497
498
499
500
501
502
503
504
import streamlit as st
from FineTuner import FineTuner
from config.conf import config
import subprocess
from time import sleep
st.set_page_config(page_title='T5 MAGIC', layout="wide")
# Header display
def display_header():
header_container = st.empty()
header_container.markdown("""
# Text Summarization Demo with T5
A fine-tuned T5 model for text summarization
""")
###########################################################################################
# Summarization display
def display_summaries(text, summaries, container):
container.empty()
container.text_area('ORIGINAL TEXT {} words'.format(len(text.split())), value=text, height=300)
for key,val in summaries.items():
container.text_area(label='{} Summary {} words'.format(key, len(val.split())), value=val, height=300)
###########################################################################################
# Summarize function for direct text summarization
def text_summary_component():
models = []
for model_name in config['model_ckpt_path'].keys():
if st.session_state['model_choice_{}'.format(model_name)]:
models.append((model_name, config['model_ckpt_path'][model_name]))
display_header()
summary_container = st.container()
text = st.session_state.text
if text and models and len(text.split(' ')) >= 50:
summaries= {}
for (model_name,model_path) in models:
tunner = FineTuner(
model_name=model_name,
pre_tuned=True,
saved_checkpoint_file_name=model_path
)
# fine tunning the model
with summary_container.info('Loading {} from Checkpoint'.format(model_name.title())):
tunner.fit_and_tune()
# summarizing with model
with summary_container.success('Summarizing {}'.format(model_name.title())):
summary = tunner.summarize(
text,
st.session_state.maximum_summary_length
)
summaries[model_name]=summary
display_summaries(
text=text,
summaries=summaries,
container=summary_container
)
else:
summary_container.error("INVALID INPUT, OR NO CHOSEN MODEL, TRY AGAIN")
sleep(3)
###########################################################################################
# display matched results
def display_matches(out):
search_res = out.split('[CEL]')
st.success('Displaying Results')
for res in search_res[:-1]:
if res[0] == "\n":
id = res[2:7].strip()
else:
id = res[1:7].strip()
st.text_area(label=id, value=res, key="matched-doc-{}-text".format(id), height=500)
st.button(
label='Summarize',
key="matched-doc-{}-btn".format(id),
on_click=text_searched_summary_component,
kwargs={'text-id' : "matched-doc-{}-text".format(id)}
)
st.write("""
---
""")
#####################################################################################
# Summarize function for searched results
def text_searched_summary_component(**kwargs):
# get available models
models = []
for model_name in config['model_ckpt_path'].keys():
if st.session_state['model_choice_{}'.format(model_name)]:
models.append((model_name, config['model_ckpt_path'][model_name]))
display_header()
summary_container = st.container()
text = st.session_state[kwargs['text-id']]
if text and models:
summaries= {}
for (model_name,model_path) in models:
tunner = FineTuner(
model_name=model_name,
pre_tuned=True,
saved_checkpoint_file_name=model_path
)
# Loading the model from checkpoint
with summary_container.info('Loading {} from Checkpoint'.format(model_name.title())):
tunner.fit_and_tune()
# Summarizing with model
with summary_container.success('Summarizing with {}'.format(model_name.title())):
summary = tunner.summarize(
text,
st.session_state.maximum_summary_length
)
summaries[model_name]=summary
display_summaries(
text=text,
summaries=summaries,
container=summary_container
)
else:
summary_container.error("INVALID INPUT, OR NO CHOSEN MODEL, TRY AGAIN")
sleep(3)
#####################################################################################
# Function for searching keywords using the search engine complied file
def text_search_component():
display_header()
# make keywords comma seprated for binary file arguments
words = st.session_state.keyword
words = words.replace(" ", ",")
print(words)
# setup the search command
cmd = '{} -d {} -k {} -m /search -w {}'.format(
config['search_engine_binary_path'][config['os-system']],
config['search_data_path'],
st.session_state.maximum_searched_results,
words
)
# search the keywords
with st.warning('Searching Through the Database'):
_, out = subprocess.getstatusoutput(cmd)
# output processing
if out == "wrong arguments":
st.error('Invalid Input')
elif out.find('[CEL]') == -1:
st.error('No Matched Results')
else: display_matches(out)
####################################################################################
# front end buttons, sliders and checkboxes
left_column_sb, right_column_sb = st.sidebar.columns(2)
left_column, right_column = st.columns(2)
# Summarization Functionality button
if left_column_sb.button(
label='Summarizing',
key='summarization-main',
help='Click for Summarization Functionality'
):
display_header()
summary_container = st.container()
text = st.text_area("Original Text", key="text", height=300)
st.button(
label='Summarize',
key='summarize',
on_click=text_summary_component,
)
# Searching Functionality button
elif right_column_sb.button(
label='Searching',
key='searching-main',
help='Click for Searching'
):
display_header()
st.text_input("Search Keyword", key="keyword")
st.button(label='Search', key='search', on_click=text_search_component)
# Home Page button
else:
md_container = st.empty()
md_container.markdown("""
# T5 Text Summarization and Searching Demo
A fine-tuned T5 model for text summarization equipped with a custom search engine
![t5-pic] (https://i.pinimg.com/736x/8e/8c/4d/8e8c4d471247b1df445a4bd11a1c8f05.jpg)
"""
)
# Model choice check boxes
st.sidebar.write("""
---
Summarization Models
""")
for model_name in config['model_ckpt_path'].keys():
st.sidebar.checkbox(
label=model_name,
value=False,
key='model_choice_{}'.format(model_name),
)
# Maximum searched results slider
st.sidebar.slider(
'Maximum Searched Results',
min_value=1,
max_value=20,
value=4,
step=1,
key='maximum_searched_results',
help='Determines the maximum number of results the search engine will display'
)
# Maximum summary length slider
st.sidebar.slider(
'Maximum Summary Length',
min_value=50,
max_value=200,
value=150,
step=10,
key='maximum_summary_length',
help='Determines the maximum summary length for the summarization of input text'
)
st.sidebar.write("""
---
""")
# shows guidelines
if st.sidebar.button(
label='Guide',
key='guide'
):
md_container = st.empty()
md_container.write(
"""
# Table of Contents
- [Table of Contents](#table-of-contents)
- [1 Features](#1-features)
- [1.1 Multiple T5 Text Summarization Models](#11-multiple-t5-text-summarization-models)
- [1.2 Single Keyword Search](#12-single-keyword-search)
- [1.3 Multiple Keyword Search](#13-multiple-keyword-search)
- [1.4 Keyword Search and Summarize](#14-keyword-search-and-summarize)
- [2 Installation/Usage](#2-installationusage)
- [3 Project Overview](#3-project-overview)
- [3.1 T5 Text Summarizer](#31-t5-text-summarizer)
- [3.1.1 T5 Background](#311-t5-background)
- [3.1.2 T5 Final Architecture](#312-t5-final-architecture)
- [3.1.3 T5 Summarization Fine-Tuning](#313-t5-summarization-fine-tuning)
- [3.2 Text Search Engine](#32-text-search-engine)
- [3.2.1 Text Dataset Architecture](#321-text-dataset-architecture)
- [3.2.2 Text Search Logic](#322-text-search-logic)
- [4 Current Issues](#4-current-issues)
- [6 Roadmap](#6-roadmap)
- [7 Acknowledgements](#7-acknowledgements)
# 1 Features
## 1.1 Multiple T5 Text Summarization Models
The `Summarizing` functionality of program allows the user to enter a text with more than 50 words in the `text_area`, choose single/multiple models to be used for summarization at once and adjust the `Maximum Summary Length`. The program will load the model from checkpoint, and summarize the text in less than a minute.
Current supported summarization models:
- t5-small
- t5-base

## 1.2 Single Keyword Search
The `Searching` functionality of the program allows the user to enter a single keyword in the search `text_input`, choose the `Maximum Searched Results` to be displayed and wait for the search engine to find the occurrences of the keyword in the news/articles dataset and display them with a custom ranking scheme dependent of the number of occurrences.

## 1.3 Multiple Keyword Search
The `Searching` functionality of the program allows the user to enter multiple keywords either in the format of `keyword_1,keyword_2>` or `<keyword_1 keyword_2>` in the search `text_input`, choose the `Maximum Searched Results` to be displayed and wait for the search engine to find the occurrences of the keyword in the news/articles dataset and display them with a custom ranking scheme dependent of the number of occurrences. (**Note:** the search engine will consider each `<keyword>` separately and not as a whole)

## 1.4 Keyword Search and Summarize
The `Searching` and `Summarizing` functionalities can also be used together by allowing the user to enter single/multiple keywords in the search `text_input`, choose the `Maximum Searched Results` to be displayed, choose single/multiple models to be used for summarization at once and adjust the `Maximum Summary Length`. Upon displaying search results, clicking on the `Summarize` button underneath the displayed results will summarize the text of the displayed document by loading the model from checkpoint and summarizing the text in less than a minute.

# 2 Installation/Usage
1. Clone the repository and change the directory to the path the cloned repository is located at
```bash
git clone https://github.com/farazkh80/SearchEngine.git
cd SearchEngine
```
2. In order to enable the search engine choose the right binary file you would need to change the operating system in **line 7** of `config/conf.py` of the repo.
(change to match running os supported options: 'win-32', 'win-64' and 'unix')
```python
config/conf.py
'os-system': 'unix', # change to match running os supported options: 'win-32', 'win-64' and 'unix'
```
3. Create a virtual environment and activate it
```bash
virtualenv venv
venv/Scripts/activate
```
4. Install the project requirements
```bash
pip install -r requirements.txt
```
1. You need to download the pre-tuned t5-model checkpoints and place them in the root of the repository (if you are willing to fine-tune from scratch and have the required computational power you can skip this step)
- [Google Drive Share Link for t5-base](https://drive.google.com/file/d/1NmQGZHbesXGQgJxO07jbcV-7dGK1MUfO/view?usp=sharing)
- [Google Drive Share Link for t5-small](https://drive.google.com/file/d/1dr_QJndLOk48I_bckIqGusr6Ok267ph0/view?usp=sharing)
6. Run the streamlit app and you have a super powerful text summarization and searching interface
```bash
streamlit run app.py
```
# 3 Project Overview
T5 Text Search and Summarize Engine has two core components, a **Transformer-Based Pre-Trained T5 Model Fine-Tuner** used for text summarization and a **Token-Based Text Searcher**.
The primary use of this project is for news text summarization, however, it can be expanded for any type of natural language summarization.
## 3.1 T5 Text Summarizer
The T5 Text Summarizer part of this project uses the pre-trained PyTorch `transformers.ioT5ForConditionalGeneration` model from the [Hugging Face API](https://huggingface.co/transformers/model_doc/t5.html) and [PyTorch Lightning](https://pytorch-lightning.readthedocs.io/en/latest/) research framework for handling cross-hardware training, model check-pointing and logging.
T5 model can be used for wide range of NLP tasks such as Translation, Summarization, Classification, Regression and Q&A, if fine-tuned with a dataset relevant to the desired task.
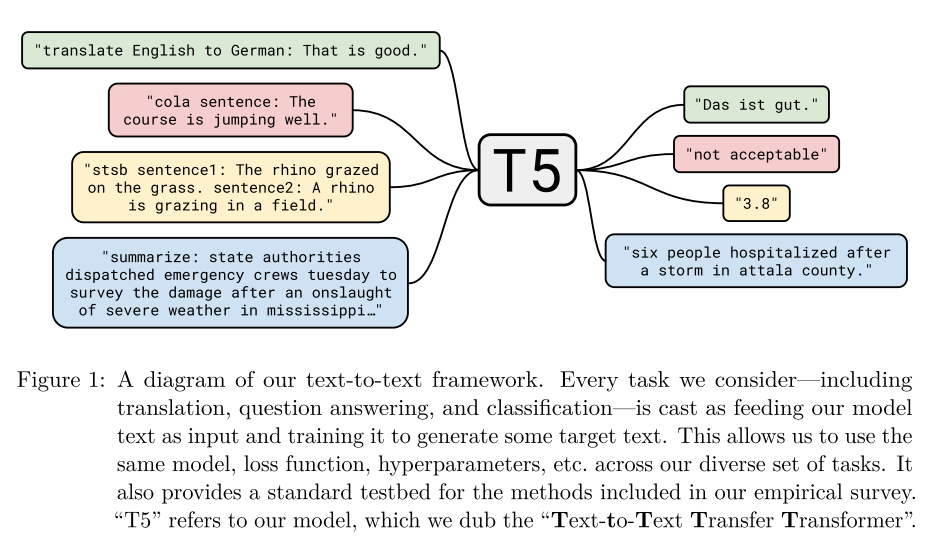
To see how T5 is able to achieve these results, a brief explanation of the [background](#311-t5-background) and [architecture](#312-t5-final-architecture) behind T5 is provided below.
### 3.1.1 T5 Background
[T5](https://arxiv.org/abs/1910.10683) (**T**ext-**t**o-**T**ext **T**ransfer **T**ransformer) model is a result of a systematic study conducted by Google researchers as presented in [Exploring the Limits of Transfer Learning with a Unified Text-to-Text Transformer](https://arxiv.org/abs/1910.10683) by Colin Raffel, Noam Shazeer, Adam Roberts, Katherine Lee, Sharan Narang, Michael Matena, Yanqi Zhou, Wei Li, Peter J. Liu.
In their study, researchers start with a baseline model roughly equivalent to the [original Transformer](https://arxiv.org/abs/1706.03762) proposed by [Vaswani et al. (2017)](https://arxiv.org/abs/1706.03762) with 3 main exceptions of removing the Layer Norm bias, placing the layer normalization outside residual path and using a different position embedding scheme.
For training, T5 uses [C4](tensorlow.text.c4) dataset, a heuristic filtered version of common crawl web extracted text. In filtering, any line that didn’t end in a terminal punctuation mark, or containing the word javascript or any pages that had a curly bracket get removed. Dataset is then deduplicated by taking a sliding window of 3 sentence chunks so that only one of them appeared. C4 ends up with roughly 750 gigabytes of clean-ish English text.
The final T5 model is obtained by **altering the model setup one aspect at a time** and **choosing the option with the best performance**.
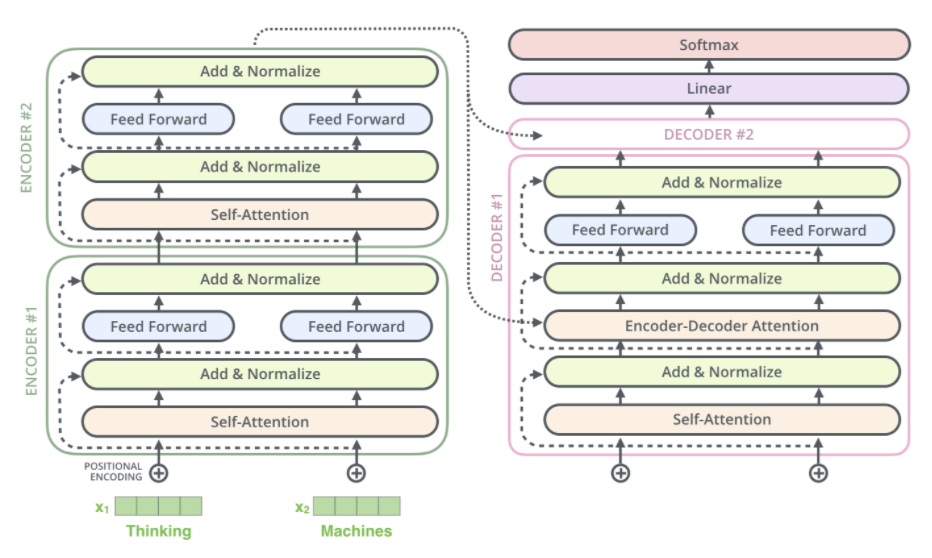
### 3.1.2 T5 Final Architecture
- **Input and Output Format** T5 uses a "text-to-text" format to provide a consistent training objective for the diverse set of tasks described in part [3.1 T5 Text Summarizer](#31-t5-text-summarizer), i.e. input is "translate English to German: That is good" and the output is "Das ist gut".
<br>
- **Objective** Finalized T5 base model's objective is the span-corruption objective with a span length of 3 and corruption rate of 15% of the original sequence, inspired by SpanBert ([Joshiet al., 2019](https://arxiv.org/abs/1907.10529))
<br>
- **Longer training** Finalized T5 base model uses a batch size of $2^{11}$ sequences of length 512 and training steps of 1 million steps, corresponding to a total of 1 trillion pre-training tokens
<br>
- **Model sizes** Finalized T5 model is available in following sizes:
- **Base.** 12-headed attention, 12 layers in each encoder and decoder with 220 million parameters
- **Small.** 8-headed attention, 6 layers in each encoder and decoder with 60 million parameters
- **Large.** 16-headed attention, 24 layers in each encoder and decoder with 770 million parameters
- **3B.** 32-headed attention 24 layers in each encoder and decoder with 2.8 billion parameters
- **11B.** 128-headed attention 24 layers in each encoder and decoder with 11 billion parameters
<br>
- **Fine-tuning** The finalized T5 model is fine-tuned with a batch size of 8 length-512 sequences for each GLUE and SuperGlue tasks with checkpoints being saved every 1,000 steps.
<br>
- **Beam Search** Finalized T5 model uses beam search with a beam width of 4 parameters and a length penalty of 0.6 for the WMT translations and CNN/DM summarization tasks.
Overall performance of finalized T5 Models is shown in the figure below.

### 3.1.3 T5 Summarization Fine-Tuning
For fine-tuning T5 for the task of news summarization, a [news_summary dataset](https://www.kaggle.com/sunnysai12345/news-summary) with +4500 news texts and summaries has been used.
Hyper-parameters used for fine-tunning:
- **For tokenization**
- text_max_token_len: 512 tokens
- summary_max_token_len: 128 tokens
- padding: "max_length"
- truncation: True
- add_special_tokens: True
<br>
- **For Fine-Tuning**
- epochs: 3
- batch_size: 8
- test_size: 0.1
<br>
- **For Summarization**
- max_length: adjustable (default=150)
- num_beams: 2
- repetition_penalty: 1.0
- length_penalty: 1.0
- early_stopping: True
Currently, only two of [Hugging Face](https://huggingface.co/transformers/model_doc/t5.html) T5 models are fine-tuned which are `t5-small` trained with GPU(PyTorch CUDA) and `t5-base` trained with Google's 8-core v2 TPU.
## 3.2 Text Search Engine
The Text Searching Engine is developed purely in `C` and `C++` for optimized speed and memory handling. The search engine is initialized with a static dataset containing thousands of news and articles rendered from the web.
After initialization, the search engine is tasked with searching for a certain word in the dataset and display the documents(news) containing the searched keyword with a custom ranking scheme.
### 3.2.1 Text Dataset Architecture
The dataset initialization is achieved by leveraging `Trie` for storing tokens (denoted as `Trienode`) and `Linked List` data structures for mapping document id to news/document text (denoted as `Mymap`) and storing times of occurrences of each token in all news/document ids(denoted as `Listnode`).

### 3.2.2 Text Search Logic
Text searching is achieved through main functionalities:
1. `/tf` : given a `<key-word>` and a document `<id>`, will search the `<keyword>` token in the `Trienode` and retrieve the times of occurrences for the corresponding `<id>` of the token's `<Listnode>`, ultimately returning the number of times the `<key-word>` has been detected in document with id `<id>`. (not supported by the web-interface)
<br>
2. `/df` : given a `<key-word>`, will leverage the `/tf` function to search through all the documents and return the total number of times the `<key-word>` has been detected in the whole dataset. (not supported by the web-interface)
<br>
3. `/search`: given a set of `<keyword1,keyword2,..>` will leverage `/tf` search to find the number of occurrences of each keyword in documents, calculate a logarithmic score for ranking scheme and store it in a list(denoted as `Scorelist`) and finally display the results in a descending order of scores which is achieved through transforming the `Scorelist` to a `Maxheap` structure, and removing highest score one at a time. (supported by the web-interface)
# 4 Current Issues
- Searching dataset initialization takes place before each new search, reducing the searching speed
- Model loading from checkpoint also happens before each summarization task
- Constant, non-configurable `text_max_token_len` of 512 and `summary_max_token_len` of 128 as suggested by the original [T5](https://arxiv.org/abs/1910.10683) paper, limit the word-range of input and output text
# 6 Roadmap
- Fixing Issues:
- Avoid searching dataset initialization from happening before each search by either running both the text summarization and searching engines in a parallel setup or storing search dataset in a database.
- Load model from checkpoint only once at the beginning of the program or just before the first summarization prompt
- Add configurable `text_max_token_len` and `summary_max_token_len` to increase text word-range flexibility.
- New Features:
- Fine-tune T5 Models with higher number of parameters such as `t5-large` and `t5-3B` for better results.
- Add other NLP models such as `BERT` and `ALBERT` for performance comparison.
- Add a web news scrapper to maintain an up-to-date version of latest most popular `n` news.
# 7 Acknowledgements
T5 Text Summarizer
- [Exploring the Limits of Transfer Learning with a Unified Text-to-Text Transformer](https://arxiv.org/abs/1910.10683)
- [Attention Is All You Need](https://arxiv.org/abs/1706.03762)
- [Hugging Face T5 API](https://huggingface.co/transformers/model_doc/t5.html)
- [PyTorch Lightning Trainer](https://pytorch-lightning.readthedocs.io/en/latest/common/trainer.html)
- [Text Summarization with T5](https://www.youtube.com/watch?v=KMyZUIraHio)
Search Engine
- [Building a Search Engine with C++](https://www.education-ecosystem.com/nikos_tsiougkranas/ljJg5-how-to-build-a-search-engine-in-c/yDd46-intro-how-to-build-a-search-engine-in-c/)
"""
)
st.sidebar.write("""
[Github](https://github.com/farazkh80/SearchEngine)
[Website](https://www.faraz-khoubisirat.com)
""")