You signed in with another tab or window. Reload to refresh your session.You signed out in another tab or window. Reload to refresh your session.You switched accounts on another tab or window. Reload to refresh your session.Dismiss alert
I've tried MOFA with several datatypes, some having ~50 samples while others have 15 or 22 samples.
Here's an overview of the data:
RNA.vst = vst transformed RNA-seq data
DNAm = DNAm m-values
metab_annot_extract = metabolites, cell extract, annotated
metab_annot_secreted = metabolites, cell secretion, annotated
metab_unannot_extract = metabolites, cell extract, unannotated
crispr_qBF = quantile normalized Bayes Factors from CRISPR screens similar to that in Hart et al. 2015, but using a smaller library.
I ran MOFA with the following training options on this data (20 other models were run, most producing similar results, none having a common axis of variation shared between all datatypes)
The resulting model has the following explained variance:
and correlattion between factors:
The results would seem to imply that metabolomics data do not share a common axis with the RNA-seq and DNA methylation data. When I run PCA on eahc of the data matrices as input to MOFA individually however, I get clean or relatively clean separation of clusters identified in RNA-seq data in each datatype:
RNA-seq:
DNA methylation:
CRISPR Screen:
Metabolites, Annotated Cell Extract:
Metabolites, Unannotated Cell Extract:
Metabolites, Annotated Secretion:
I would have expected a common factor to be found for all datatypes, but I'm wondering if there's too much missing data here.
Hi @whitleyo ,
it is an interesting problem. Based on the PCA results, the separation is more clear in some data types than in others, so it is not be surprising if MOFA does not capture a factor that is common across all data types. But it should definitely capture a factor that separates the groups that you are considering. Can you check if this is the case?
P.S. Please upgrade to MOFA2 (https://github.com/bioFAM/MOFA2), it is a better software and we are no longer maintaining this repository. Also, feel free to join the Slack group where we can have a more interactive discussion.
Hi,
I've tried MOFA with several datatypes, some having ~50 samples while others have 15 or 22 samples.
Here's an overview of the data:
RNA.vst = vst transformed RNA-seq data
DNAm = DNAm m-values
metab_annot_extract = metabolites, cell extract, annotated
metab_annot_secreted = metabolites, cell secretion, annotated
metab_unannot_extract = metabolites, cell extract, unannotated
crispr_qBF = quantile normalized Bayes Factors from CRISPR screens similar to that in Hart et al. 2015, but using a smaller library.
I ran MOFA with the following training options on this data (20 other models were run, most producing similar results, none having a common axis of variation shared between all datatypes)
The resulting model has the following explained variance:
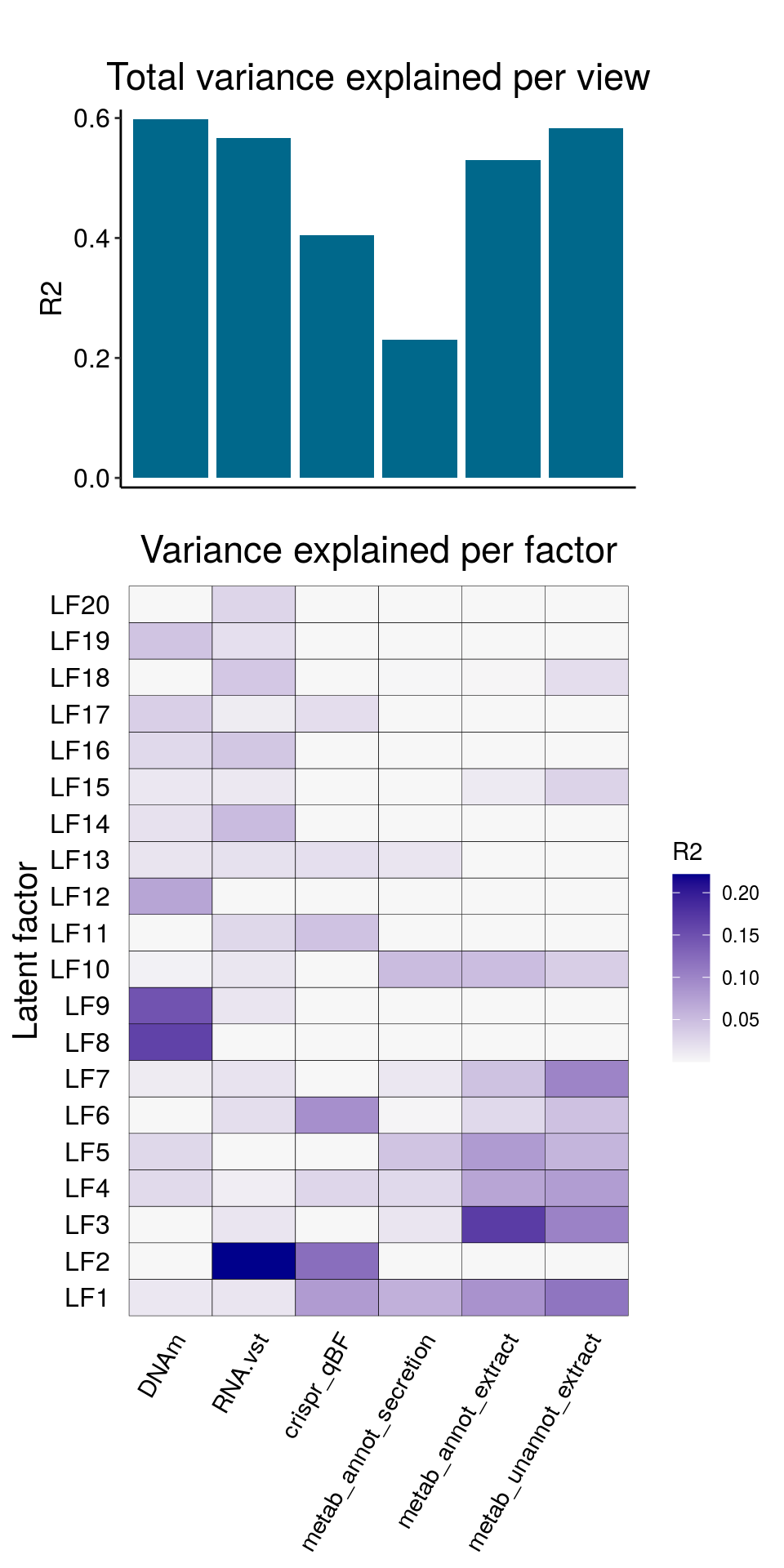
and correlattion between factors:
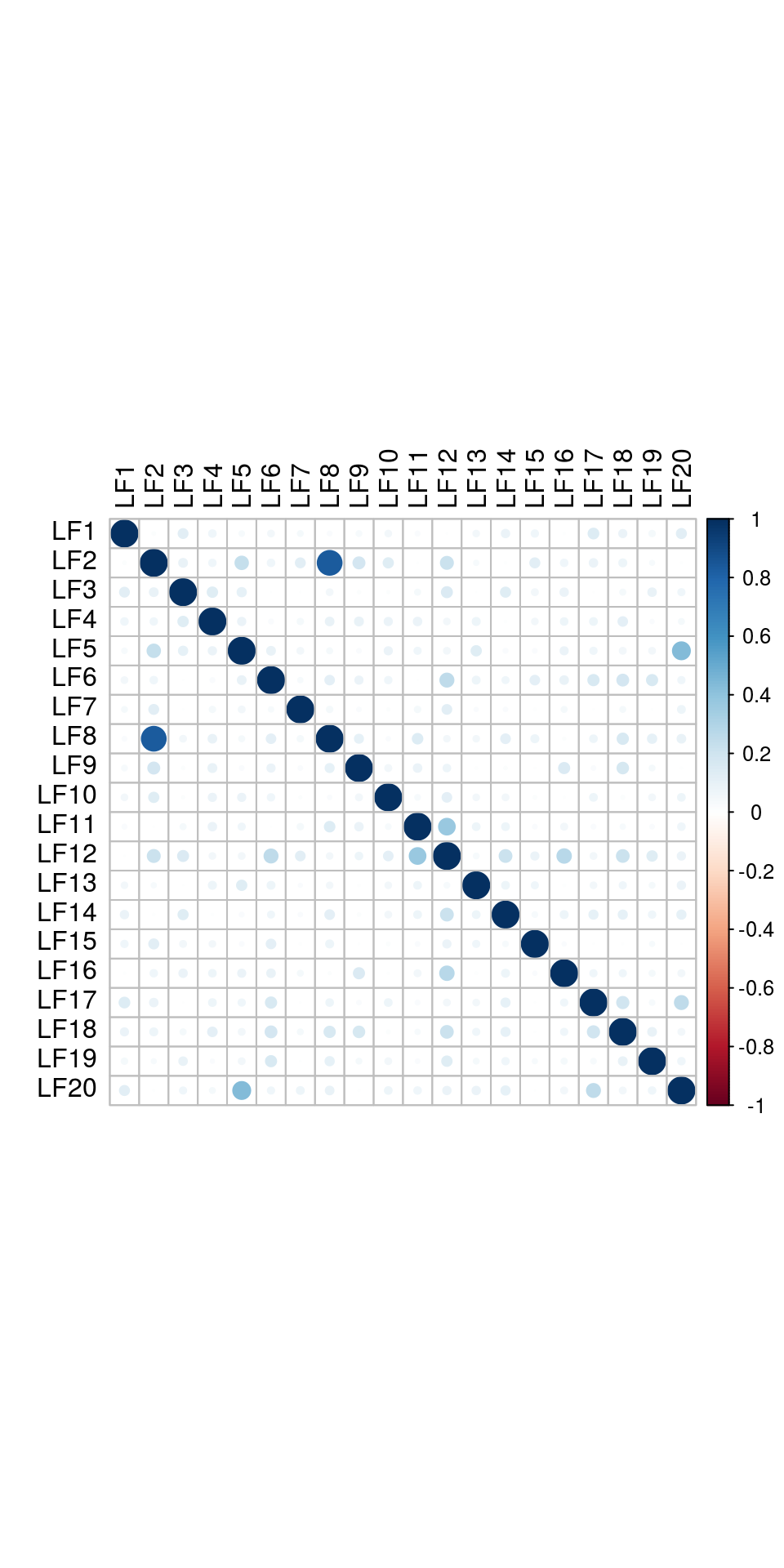
The results would seem to imply that metabolomics data do not share a common axis with the RNA-seq and DNA methylation data. When I run PCA on eahc of the data matrices as input to MOFA individually however, I get clean or relatively clean separation of clusters identified in RNA-seq data in each datatype:
RNA-seq:
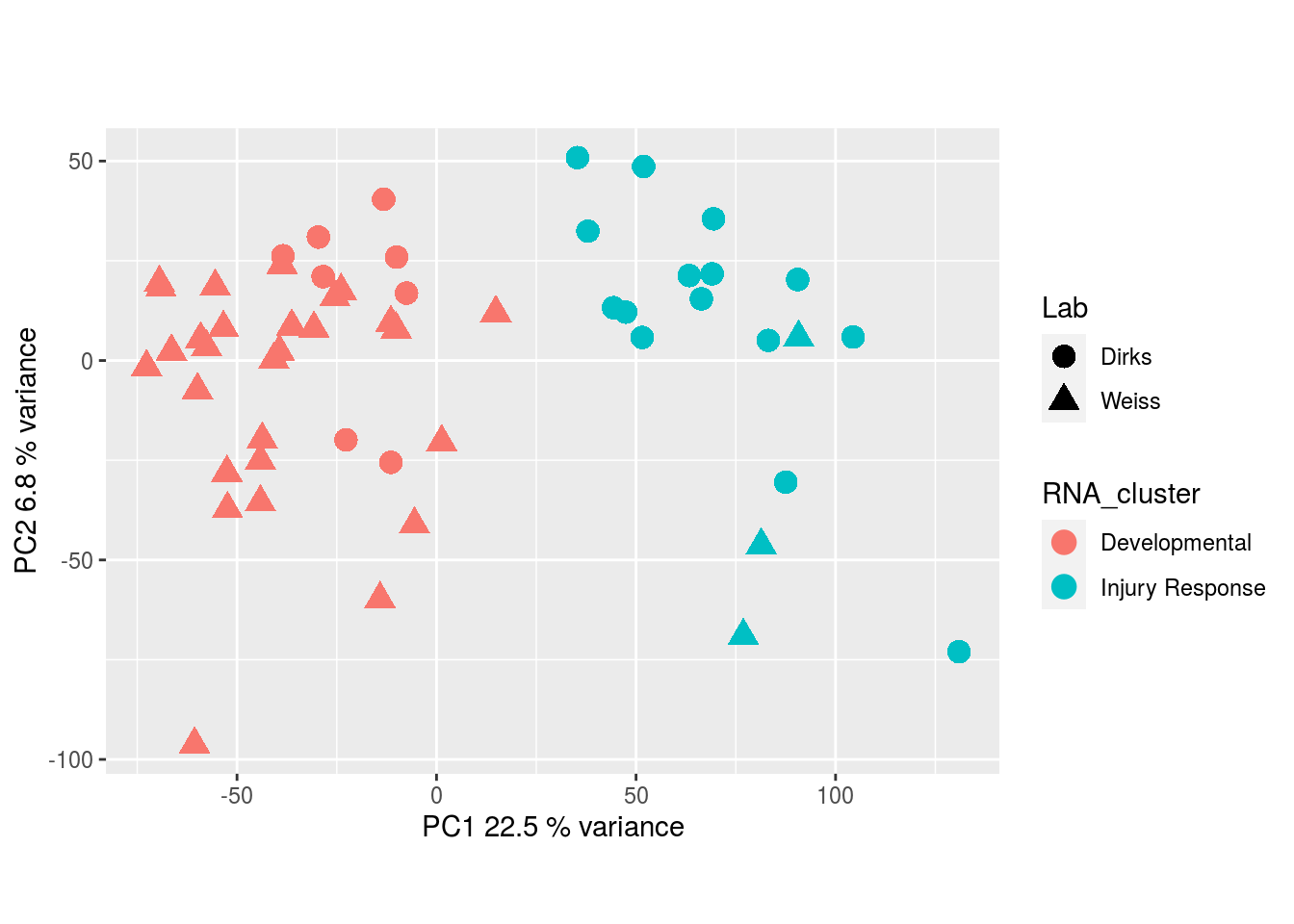
DNA methylation:
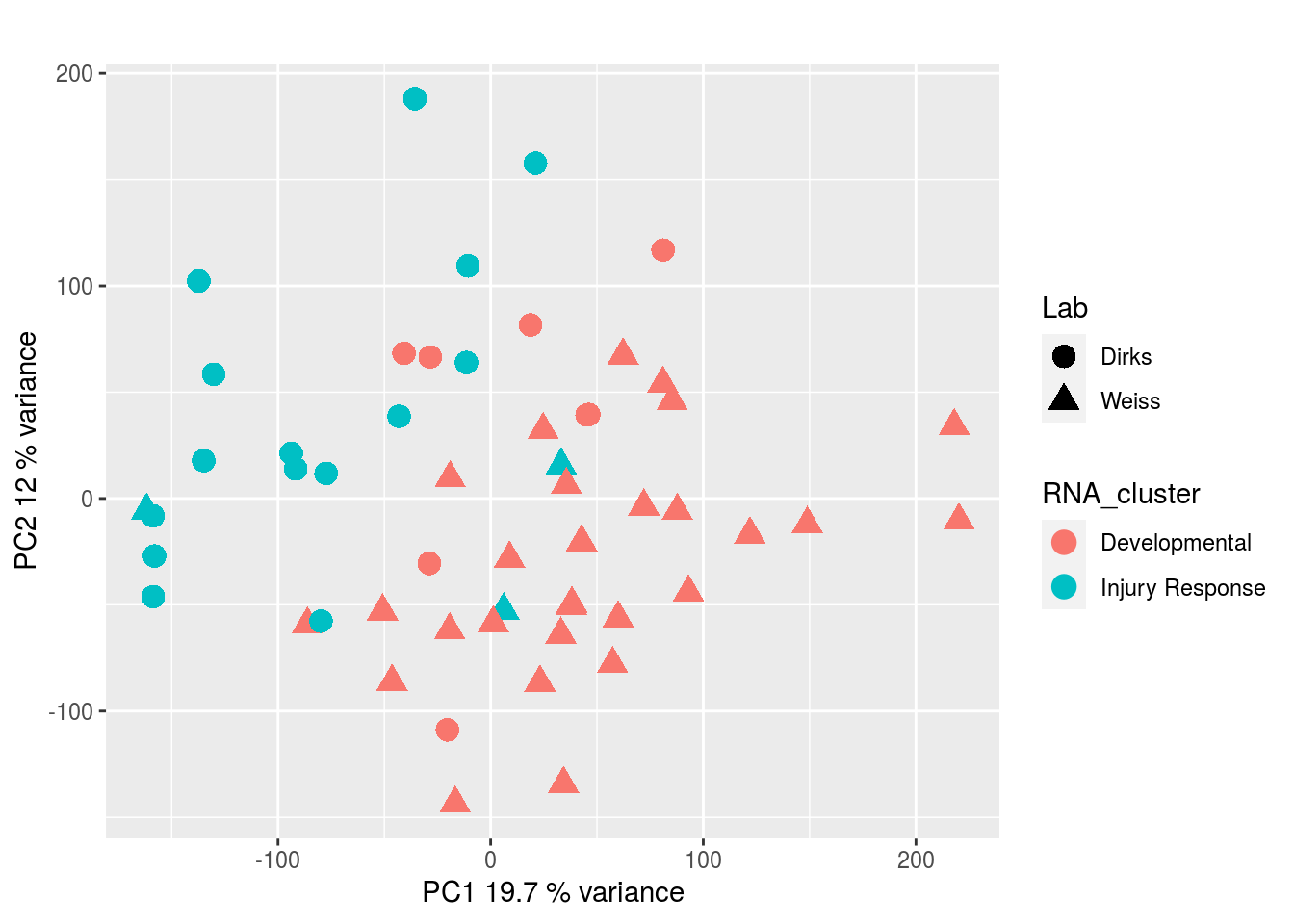
CRISPR Screen:
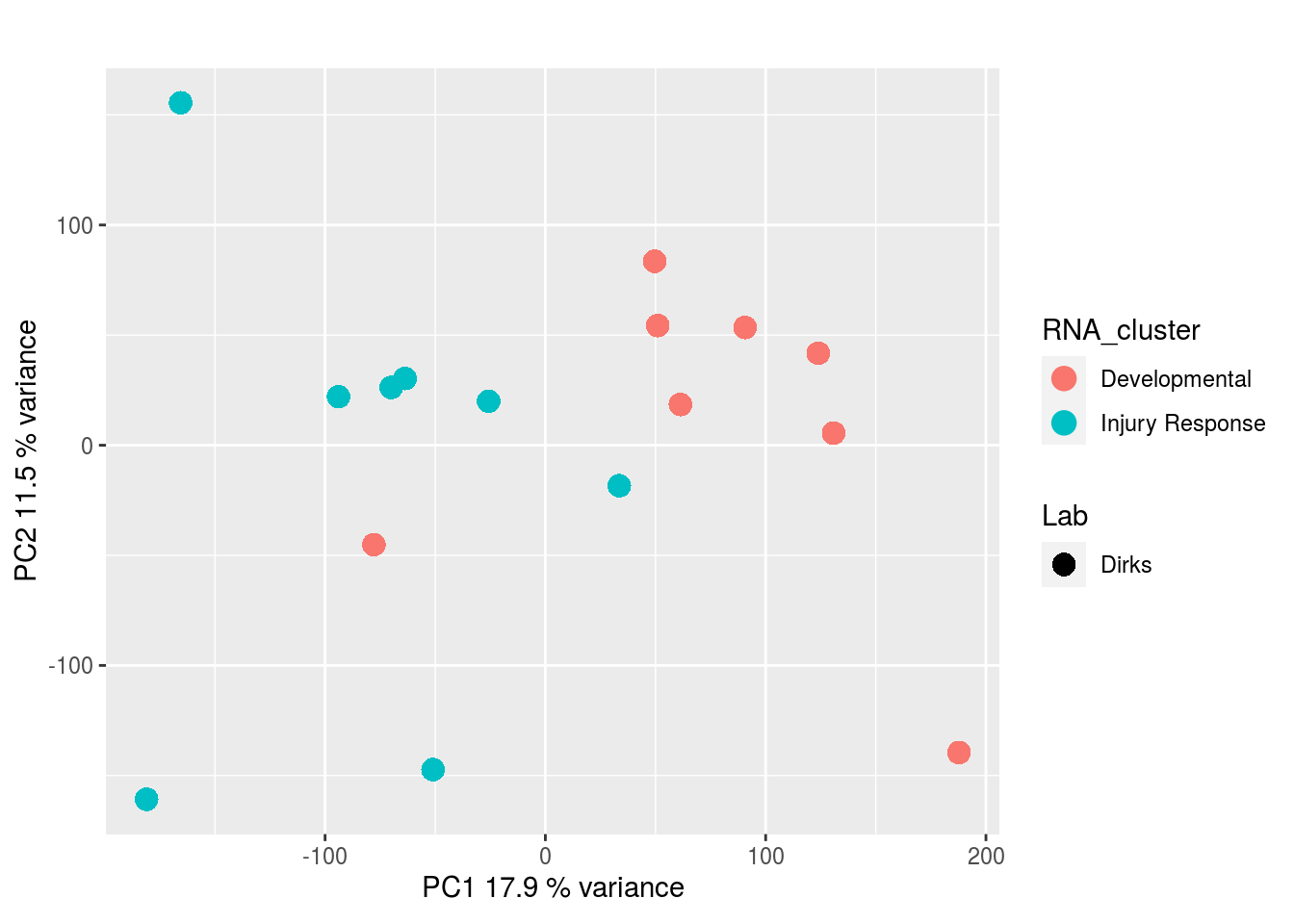
Metabolites, Annotated Cell Extract:
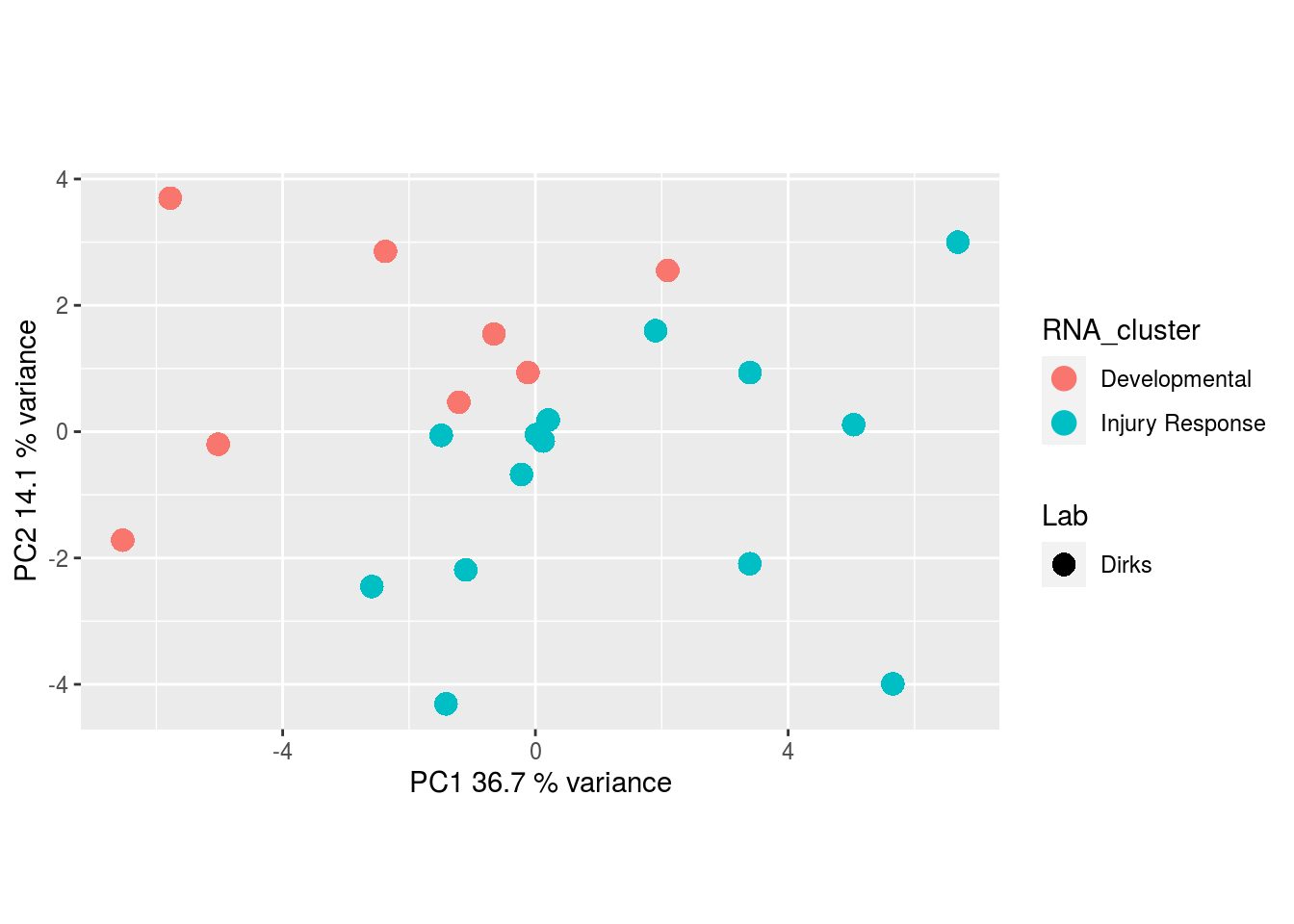
Metabolites, Unannotated Cell Extract:
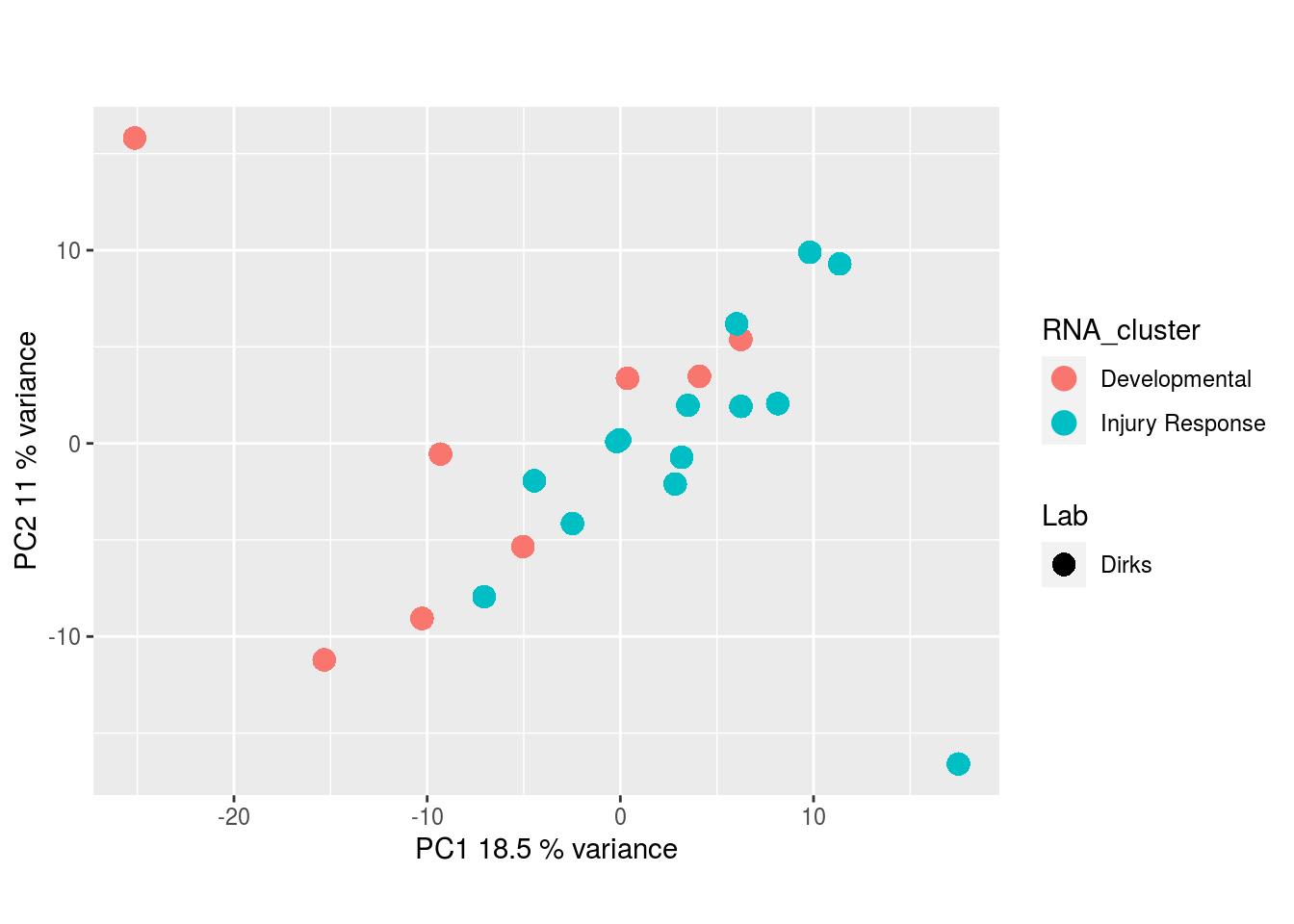
Metabolites, Annotated Secretion:
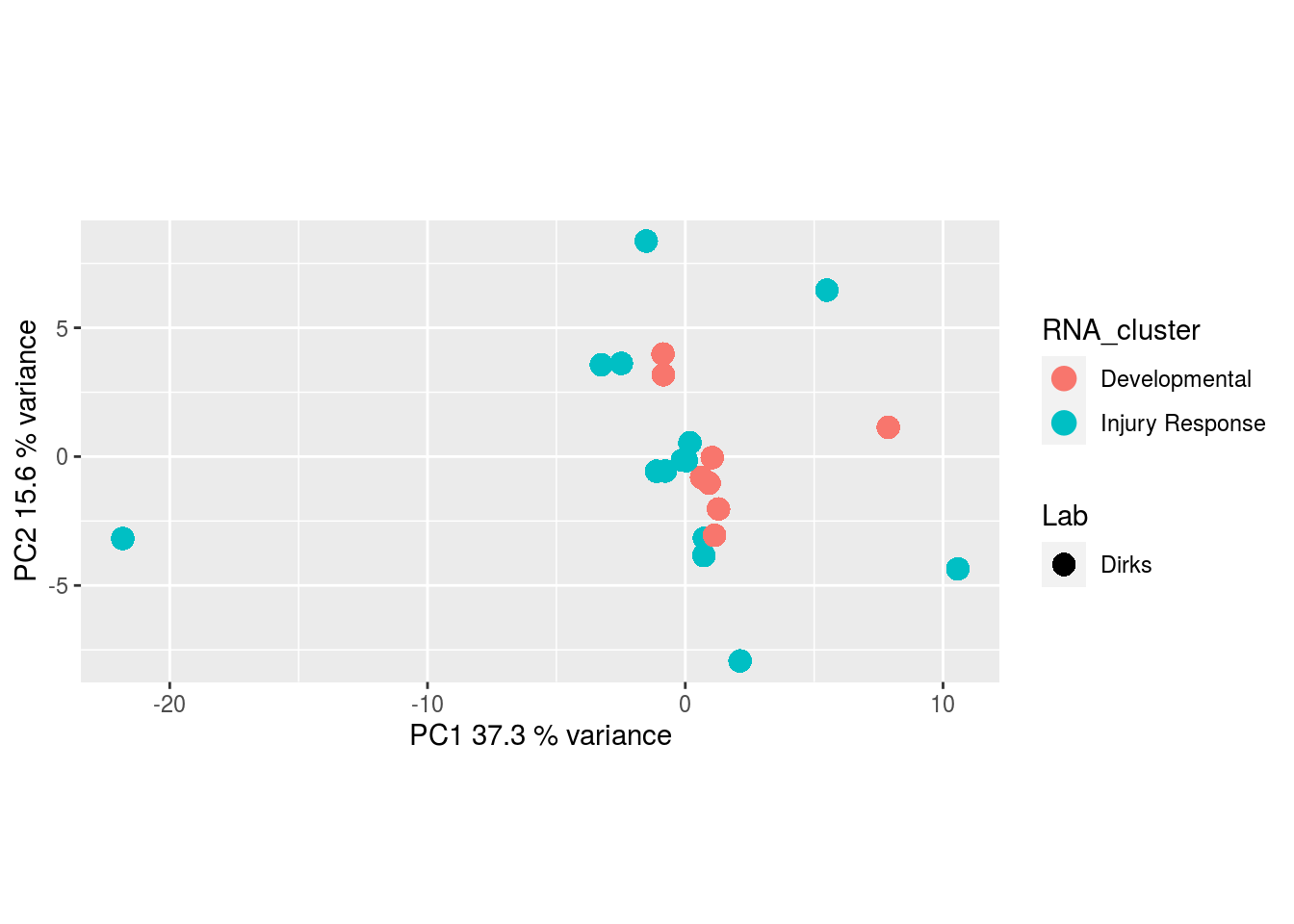
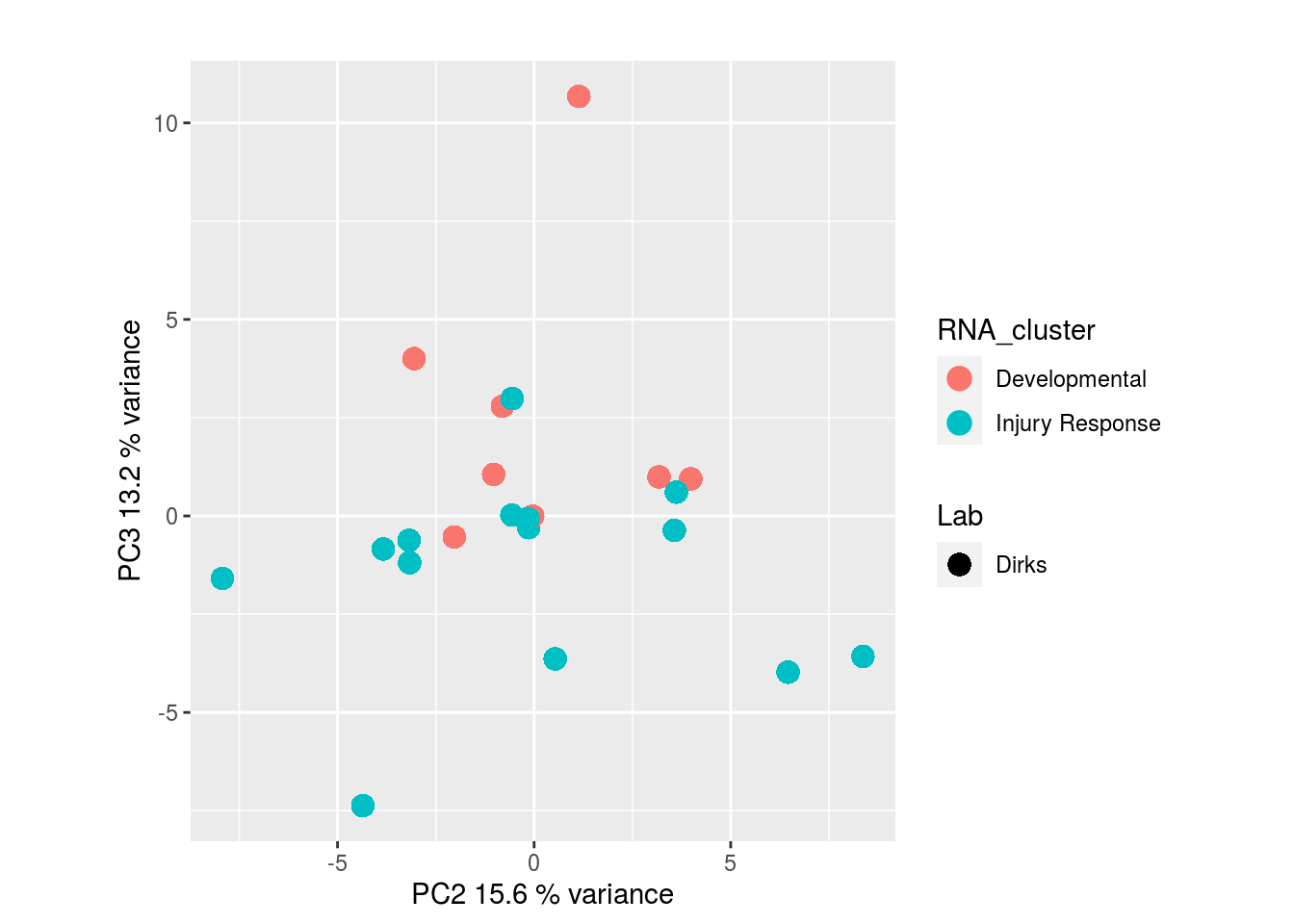
I would have expected a common factor to be found for all datatypes, but I'm wondering if there's too much missing data here.
Session Info:
The text was updated successfully, but these errors were encountered: