-
Notifications
You must be signed in to change notification settings - Fork 3
/
Copy pathapp.py
332 lines (242 loc) · 14.6 KB
/
app.py
1
2
3
4
5
6
7
8
9
10
11
12
13
14
15
16
17
18
19
20
21
22
23
24
25
26
27
28
29
30
31
32
33
34
35
36
37
38
39
40
41
42
43
44
45
46
47
48
49
50
51
52
53
54
55
56
57
58
59
60
61
62
63
64
65
66
67
68
69
70
71
72
73
74
75
76
77
78
79
80
81
82
83
84
85
86
87
88
89
90
91
92
93
94
95
96
97
98
99
100
101
102
103
104
105
106
107
108
109
110
111
112
113
114
115
116
117
118
119
120
121
122
123
124
125
126
127
128
129
130
131
132
133
134
135
136
137
138
139
140
141
142
143
144
145
146
147
148
149
150
151
152
153
154
155
156
157
158
159
160
161
162
163
164
165
166
167
168
169
170
171
172
173
174
175
176
177
178
179
180
181
182
183
184
185
186
187
188
189
190
191
192
193
194
195
196
197
198
199
200
201
202
203
204
205
206
207
208
209
210
211
212
213
214
215
216
217
218
219
220
221
222
223
224
225
226
227
228
229
230
231
232
233
234
235
236
237
238
239
240
241
242
243
244
245
246
247
248
249
250
251
252
253
254
255
256
257
258
259
260
261
262
263
264
265
266
267
268
269
270
271
272
273
274
275
276
277
278
279
280
281
282
283
284
285
286
287
288
289
290
291
292
293
294
295
296
297
298
299
300
301
302
303
304
305
306
307
308
309
310
311
312
313
314
315
316
317
318
319
320
321
322
323
324
325
326
327
328
329
330
331
332
import streamlit as st
nav = st.sidebar.radio("Navigate",["Home", "Classify"])
if nav == "Home":
if __name__ == "__main__":
st.title('Music Genre Classification')
'''
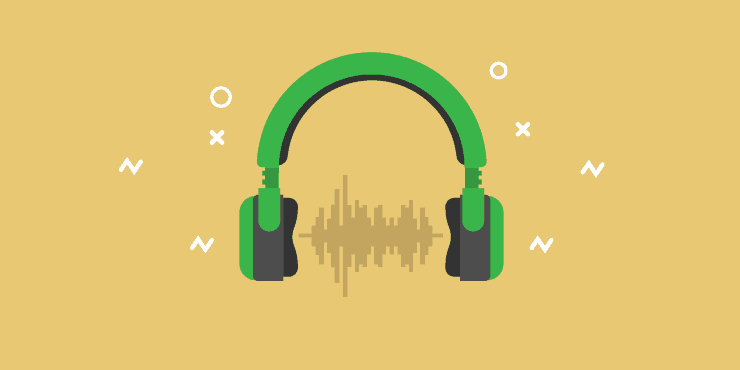
'''
if nav == "Code":
st.title('Code')
with st.echo():
# Import required libraries
import pandas as pd
import numpy as np
import matplotlib.pyplot as plt
import seaborn as sns
'''
## 1. Importing the data
'''
with st.echo():
# Read in track metadata with genre labels
tracks = pd.read_csv('fma-rock-vs-hiphop.csv')
# Read in track metrics with the features
echonest_metrics = pd.read_json('echonest-metrics.json',precise_float=True)
# Merge the relevant columns of tracks and echonest_metrics
echo_tracks = echonest_metrics.merge(tracks[['track_id','genre_top']],on='track_id')
# Inspect the resultant dataframe
echo_tracks.info()
st.text("Int64Index: 4802 entries, 0 to 4801\nData columns (total 10 columns):\n # Column Non-Null Count Dtype\n--- ------ -------------- -----\n 0 track_id 4802 non-null int64\n 1 acousticness 4802 non-null float64\n 2 danceability 4802 non-null float64\n 3 energy 4802 non-null float64\n 4 instrumentalness 4802 non-null float64\n 5 liveness 4802 non-null float64\n 6 speechiness 4802 non-null float64\n 7 tempo 4802 non-null float64\n 8 valence 4802 non-null float64\n 9 genre_top 4802 non-null object\ndtypes: float64(8), int64(1), object(1)\nmemory usage: 412.7+ KB")
'''
## 2. Pairwise relationships between continuous variables
We want to avoid using variables that have strong correlations with each other, hence avoiding feature redundancy
'''
with st.echo():
# Create a correlation matrix
corr_metrics = echo_tracks.corr()
corr_metrics.style.background_gradient()
st.write(corr_metrics.style.background_gradient())
'''
## 3. Normalizing the feature data
'''
with st.echo():
# Define our features
features = echo_tracks.drop(['genre_top','track_id'],axis=1)
# Define our labels
labels = echo_tracks['genre_top']
# Import the StandardScaler
from sklearn.preprocessing import StandardScaler
# Scale the features and set the values to a new variable
scaler = StandardScaler()
scaled_train_features = scaler.fit_transform(features)
with st.echo():
# %matplotlib inline
# Import our plotting module, and PCA class
import matplotlib.pyplot as plt
from sklearn.decomposition import PCA
# Get our explained variance ratios from PCA using all features
pca = PCA()
pca.fit(scaled_train_features)
exp_variance = pca.explained_variance_ratio_
# plot the explained variance using a barplot
fig, ax = plt.subplots(figsize=(9, 3))
ax.bar(range(pca.n_components_),exp_variance)
ax.set_xlabel('Principal Component')
st.write(fig)
with st.echo():
# Import numpy
import numpy as np
# Calculate the cumulative explained variance
cum_exp_variance = np.cumsum(exp_variance)
# get n_components
n_components = ((np.where(cum_exp_variance > 0.9))[0][0])
# Perform PCA with the chosen number of components and project data onto components
pca = PCA(n_components, random_state=10)
pca.fit(scaled_train_features)
pca_projection = pca.transform(scaled_train_features)
'''
## 4. Train a decision tree to classify genre
Here, we will be using a simple algorithm known as a decision tree. Decision trees are rule-based classifiers that take in features and follow a 'tree structure' of binary decisions to ultimately classify a data point into one of two or more categories. '''
with st.echo():
# Import train_test_split function and Decision tree classifier
from sklearn.model_selection import train_test_split
from sklearn.tree import DecisionTreeClassifier
# Split our data
train_features, test_features, train_labels, test_labels = \
train_test_split(pca_projection, labels,random_state=10)
# Train our decision tree
tree = DecisionTreeClassifier(random_state=10)
tree.fit(train_features,train_labels)
# Predict the labels for the test data
pred_labels_tree = tree.predict(test_features)
'''
## 5. Compare our decision tree to a logistic regression
It's always a worthwhile idea to at least test a few other algorithms and find the one that's best for our data.
'''
with st.echo():
# Import LogisticRegression
from sklearn.linear_model import LogisticRegression
# Train our logistic regression and predict labels for the test set
logreg = LogisticRegression(random_state=10)
logreg.fit(train_features,train_labels)
pred_labels_logit = logreg.predict(test_features)
# Create the classification report for both models
from sklearn.metrics import classification_report
class_rep_tree = classification_report(test_labels,pred_labels_tree)
class_rep_log = classification_report(test_labels,pred_labels_logit)
print("Decision Tree: \n", class_rep_tree)
print("Logistic Regression: \n", class_rep_log)
st.text("Decision Tree:\n precision recall f1-score support\n\n Hip-Hop 0.60 0.60 0.60 235\n Rock 0.90 0.90 0.90 966\n\n accuracy 0.84 1201\n macro avg 0.75 0.75 0.75 1201\nweighted avg 0.84 0.84 0.84 1201\n\nLogistic Regression:\n precision recall f1-score support\n\n Hip-Hop 0.77 0.54 0.64 235\n Rock 0.90 0.96 0.93 966\n\n accuracy 0.88 1201\n macro avg 0.83 0.75 0.78 1201\nweighted avg 0.87 0.88 0.87 1201")
'''
## 6. Balance our data for greater performance
Looking at our classification report, we can see that rock songs are fairly well classified, but hip-hop songs are disproportionately misclassified as rock songs.
We see that we have far more data points for the rock classification than for hip-hop. This also tells us that most of our model's accuracy is driven by its ability to classify just rock songs, which is less than ideal.
'''
with st.echo():
# Subset only the hip-hop tracks, and then only the rock tracks
hop_only = echo_tracks.loc[echo_tracks['genre_top'] == 'Hip-Hop']
rock_only = echo_tracks.loc[echo_tracks['genre_top'] == 'Rock']
# sample the rocks songs to be the same number as there are hip-hop songs
rock_only = rock_only.sample(n=len(hop_only),random_state=10)
# concatenate the dataframes rock_only and hop_only
rock_hop_bal = pd.concat([hop_only,rock_only])
# The features, labels, and pca projection are created for the balanced dataframe
features = rock_hop_bal.drop(['genre_top', 'track_id'], axis=1)
labels = rock_hop_bal['genre_top']
pca_projection = pca.fit_transform(scaler.fit_transform(features))
# Redefine the train and test set with the pca_projection from the balanced data
train_features, test_features, train_labels, test_labels = \
train_test_split(pca_projection,labels,random_state=10)
'''
## 7. Does balancing our dataset improve model bias?
We've now balanced our dataset, but in doing so, we've removed a lot of data points that might have been crucial to training our models. Let's test to see if balancing our data improves model bias towards the "Rock" classification while retaining overall classification performance.
'''
with st.echo():
# Train our decision tree on the balanced data
tree = DecisionTreeClassifier(random_state=10)
tree.fit(train_features,train_labels)
pred_labels_tree = tree.predict(test_features)
# Train our logistic regression on the balanced data
logreg = LogisticRegression(random_state=10)
logreg.fit(train_features,train_labels)
pred_labels_logit = logreg.predict(test_features)
# Compare the models
print("Decision Tree: \n", classification_report(test_labels,pred_labels_tree))
print("Logistic Regression: \n", classification_report(test_labels,pred_labels_logit))
st.text("Decision Tree: \n precision recall f1-score support\n\n Hip-Hop 0.76 0.80 0.78 225\n Rock 0.79 0.75 0.77 230\n\n accuracy 0.77 455\n macro avg 0.77 0.77 0.77 455\nweighted avg 0.77 0.77 0.77 455\n\nLogistic Regression: \n precision recall f1-score support\n\n Hip-Hop 0.83 0.80 0.82 225\n Rock 0.81 0.84 0.83 230\n\n accuracy 0.82 455\n macro avg 0.82 0.82 0.82 455\nweighted avg 0.82 0.82 0.82 455")
'''
## 8. Using cross-validation to evaluate our models
Balancing our data has removed bias towards the more prevalent class. To get a good sense of how well our models are actually performing, we can apply **cross-validation** (CV).
We will use **K-fold** CV here. K-fold first splits the data into K different, equally sized subsets. Then, it iteratively uses each subset as a test set while using the remainder of the data as train sets. Finally, we can then aggregate the results from each fold for a final model performance score.
'''
with st.echo():
from sklearn.model_selection import KFold, cross_val_score
# Set up our K-fold cross-validation
kf = KFold(n_splits=10)
tree = DecisionTreeClassifier(random_state=10)
logreg = LogisticRegression(random_state=10)
# Train our models using KFold cv
tree_score = cross_val_score(tree,pca_projection,labels,cv=kf)
logit_score = cross_val_score(logreg,pca_projection,labels,cv=kf)
with st.echo():
# Print the mean of each score
print("Decision Tree:", np.mean(tree_score), "Logistic Regression:", np.mean(logit_score))
st.text("Decision Tree: 0.7489010989010989 Logistic Regression: 0.782967032967033")
'''
## 9. Using Random Forest to increase performance
Random forests or random decision forests are an ensemble learning method for classification, regression and other tasks that operates by constructing a multitude of decision trees at training time and outputting the class that is the mode of the classes or mean/average prediction of the individual trees.
'''
with st.echo():
from sklearn.ensemble import RandomForestClassifier
from sklearn.model_selection import KFold, cross_val_score
# Set up our K-fold cross-validation
kf = KFold(n_splits=10)
rfr = RandomForestClassifier(random_state=10)
# Train our models using KFold cv
rfr_score = cross_val_score(rfr,pca_projection,labels,cv=kf)
print("Random Forest:", np.mean(rfr_score))
st.text("Random Forest: 0.8131868131868132")
if nav == "Classify":
# Importing required libraries
import pandas as pd
import numpy as np
import matplotlib.pyplot as plt
import seaborn as sns
# Read in track metadata with genre labels
tracks = pd.read_csv('fma-rock-vs-hiphop.csv')
# Read in track metrics with the features
echonest_metrics = pd.read_json('echonest-metrics.json',precise_float=True)
# Merge the relevant columns of tracks and echonest_metrics
echo_tracks = echonest_metrics.merge(tracks[['track_id','genre_top']],on='track_id')
# Inspect the resultant dataframe
echo_tracks.info()
# Define our features
features = echo_tracks.drop(['genre_top','track_id'],axis=1)
# Define our labels
labels = echo_tracks['genre_top']
# Import the StandardScaler
from sklearn.preprocessing import StandardScaler
# Scale the features and set the values to a new variable
scaler = StandardScaler()
scaled_train_features = scaler.fit_transform(features)
# Subset only the hip-hop tracks, and then only the rock tracks
hop_only = echo_tracks.loc[echo_tracks['genre_top'] == 'Hip-Hop']
rock_only = echo_tracks.loc[echo_tracks['genre_top'] == 'Rock']
# sample the rocks songs to be the same number as there are hip-hop songs
rock_only = rock_only.sample(n=len(hop_only),random_state=10)
# concatenate the dataframes rock_only and hop_only
rock_hop_bal = pd.concat([hop_only,rock_only])
# The features, labels, and pca projection are created for the balanced dataframe
features = rock_hop_bal.drop(['genre_top', 'track_id'], axis=1)
labels = rock_hop_bal['genre_top']
from sklearn.ensemble import RandomForestClassifier
from sklearn.model_selection import KFold, cross_val_score
# Set up our K-fold cross-validation
kf = KFold(n_splits=10)
rfr = RandomForestClassifier(random_state=10)
rfr.fit(features,labels)
import spotipy
from spotipy.oauth2 import SpotifyClientCredentials
def getFeatures(url):
CLIENT_ID = "a79057ae61b94921b3a987b7158e91a4"
CLIENT_SECRET = "2f3534b6441f460098c69b3213ae5258"
sp = spotipy.Spotify(auth_manager=SpotifyClientCredentials(client_id=CLIENT_ID,
client_secret=CLIENT_SECRET))
# Features = ['acousticness', 'danceability', 'energy', 'instrumentalness',
# 'liveness', 'speechiness', 'tempo', 'valence']
results = sp.audio_features(tracks = [url])
data_json = results[0]
data = [data_json['acousticness'], data_json['danceability'], data_json['energy'], data_json['instrumentalness'], data_json['liveness'], data_json['speechiness'], data_json['tempo'], data_json['valence']]
return data
'''
# Enter Spotify Track URL
#### Choose either Hip-Hop or Rock track
'''
url = st.text_input("","Paste Here")
try:
dt = getFeatures(url)
res = rfr.predict_proba([dt])
st.success(f"Hip-Hop : {100 * res[0][0]} %")
st.success(f"Rock : {100 * res[0][1]} %")
except Exception:
pass